Introduction
On the 13th of January 2019, I developed, a closed-source private neural network aimbot for the game Counter-Strike: Global Offensive. This project utilized object detection and OpenCV for autonomous aiming at enemies with minimal anti-cheat detection vectors. It was done to explore artificial intelligence and dedicated hardware processors for AI applications, specifically the Intel Neural Compute Stick 2.0.
Project Goals
While previous similar projects achieved exceptional results using high-performance GPUs such as the Nvidia GTX 1080, these solutions were limited to high-end computers. This project aimed to circumvent these restrictions by using the Intel Neural Compute Stick, eliminating the need for a dedicated GPU while maintaining high accuracy, speed, and performance. This method would allow for a "Black Box" system that could easily plug into a setup and provide quick and accurate enemy detection and termination.
Model Selection
We needed a lightweight, reliable, and accurate object-detection model. After benchmarking multiple neural network models on a GPU setup, we considered the following options:
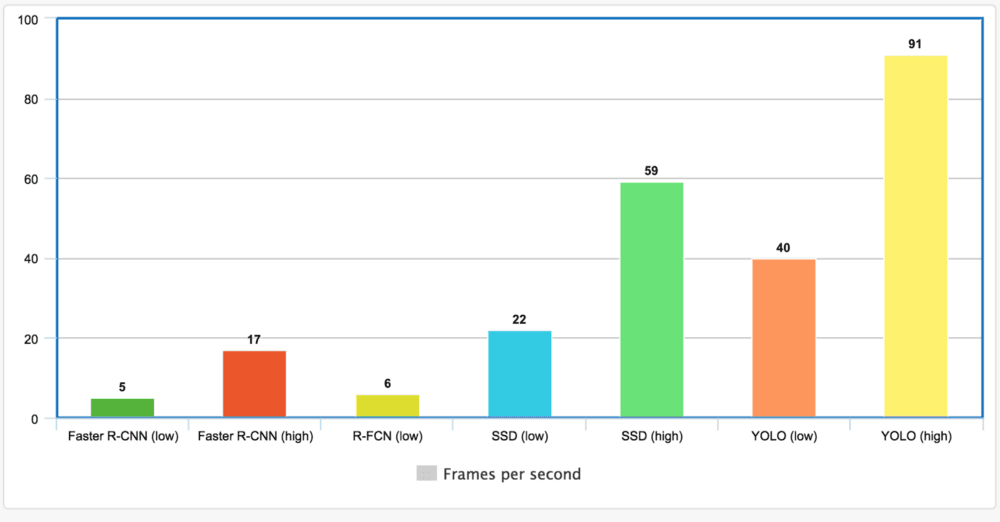
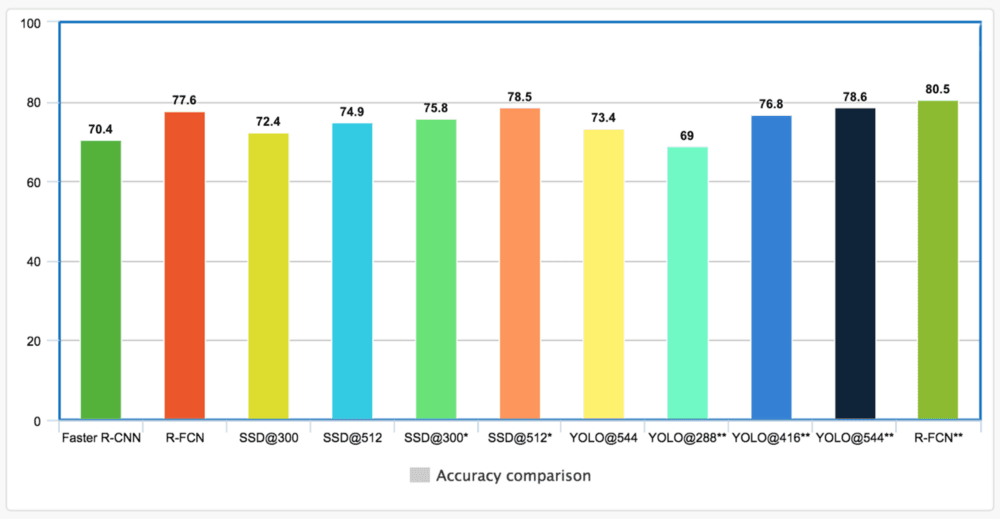
After comparing both speed and accuracy, and removing models incompatible with the Movidius neural compute stick, two main options remained: YoloV3 and MobileNetSSD.
- YoloV3: Fast but less accurate with increased false positives at longer ranges.
- MobileNetSSD: Slower but higher accuracy at increased ranges.
Implementation
After evaluation, I chose the MobileNetSSD model. I optimized the software and implemented multi-threading to increase speed and streamline processes. By creating three main pipelines (image grabbing, processing, and outputting), I improved performance by about 35%.
Two main bottlenecks were identified:
- Processing time.
- Image capturing time.
Initially, I used the pillow
library for periodic screenshots, which was inefficient. Switching to the mss
library provided significant performance benefits. Additionally, I reduced the framerate and optimized image processing by downscaling the resolution from 1920x1080 to 480x270 and converting from RGB to BGR.
Through these techniques, performance improved from 15 frames per second to 75-90 frames per second on an Intel i7 8 GB Surface Pro 4, using only the Intel Movidius Neural Compute 2.0 stick.
Conclusion
The project was an immense success. Although AI is not the ideal method for developing game hacks, it offers a unique approach. Despite the limitations, such hacks are effective and akin to early color aimbots.
Note: This project was conducted from a purely research standpoint. The source code for this hack is closed-source and unavailable upon request to protect the integrity of Counter-Strike: Global Offensive and other games.